The Profiling capability from Thermo Scientific™ SampleManager™ LIMS software provides an innovative way for laboratories to predict test results using historical data and novel machine learning (ML)-based techniques.
Anticipating the result of a test without conducting it has several benefits for a lab:
- Identify and eliminate the need to perform redundant tests.
- Reduce the number of samples tested.
- Save money by not using expensive reagents and consumables.
- Generate a prediction to all eligible samples without any extra cost or time.
- Take actions by anticipating the result of the test.
- Fail samples early that would not go on to pass the test.
The Profiling capability of the Data Analytics Solutions has many potential applications. For example, a food and beverage company might apply the Profiling capability to enable supervised learning in the food production process. In this case, SampleManager LIMS would use historical data to gain an understanding of the critical variables that determine whether a product is safe for consumers. This holistic approach considers not only the values of the individual critical variables themselves, but also the relationships between them. If a sample were to be flagged as failing, the system would alert stakeholders in advance to issue adjustments or investigations to avoid any risk to finished products.
Noticing early that a product will fail one or more tests on the production line is vital to avoid spending resources on further testing and to take corrective actions to a product to avoid failing in the future.
The Profiling capability also gives the laboratory a powerful tool to identify the tests that are more relevant based on their predictive power of an eventual pass or fail of a specific test of interest. This information will help the laboratory modify its production line to start with the more relevant tests, avoid executing a test and reduce its operational costs.
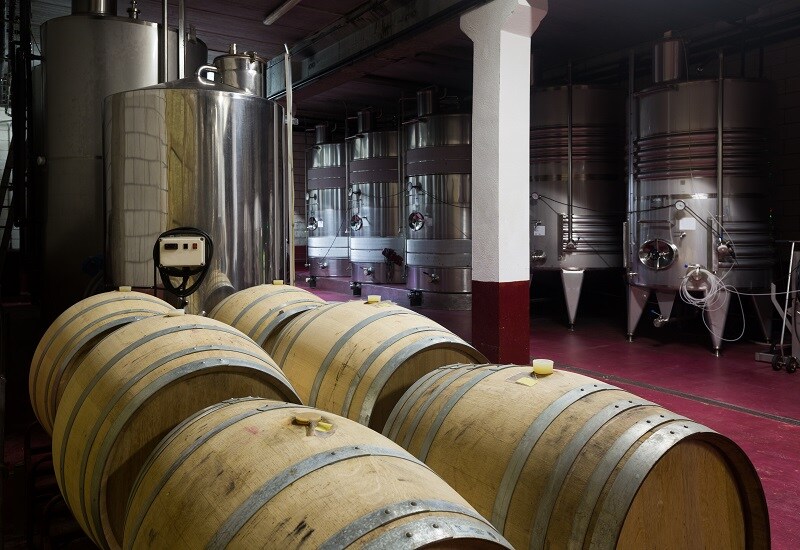
Machine learning applications in wine quality testing.
Profiling capability: A wine quality use case
To understand the benefit of the Profiling capability, consider its application in a winery laboratory. A wine production facility conducts various tests on wine samples. The tests evaluate pH, alcohol, density, residual sugars, and others. After performing the full battery of standard tests, the quality control team executes a time consuming and expensive “Quality Test” that classifies a wine as high or low quality. High quality wines are packed and sold to final customers, while low quality wines are reprocessed and tested again to assure a high-quality product. Wines that fail to pass the second quality control will be sold at a lower cost to a different customer.
Early identification of wines for reprocessing reduces costs
As the final “Quality Test” is expensive and time consuming, wine testing laboratories want to predict the outcome of this test in advance. Knowing which samples are likely to pass the “Quality Test” would help accelerate the process and save resources by avoiding the final test for products that are not likely to pass. The Profiling capability can make such predictions by analyzing complex relationships between previous tests done to the wine such as pH, alcohol, residual sugars, and others.
With this in mind, a wine laboratory could apply the Profiling capability to the wine samples and evaluate its results to consider using the model as a predictor for the final test.

Prediction of the “Quality Test” using all previous tests in the production line.
In this example, the laboratory would notice the algorithm performance meets their needs and therefore could be used to predict the results of the “Quality Test.” In case there is a high probability of failure, further testing would be stopped, and the wine would be sent to reprocessing immediately.
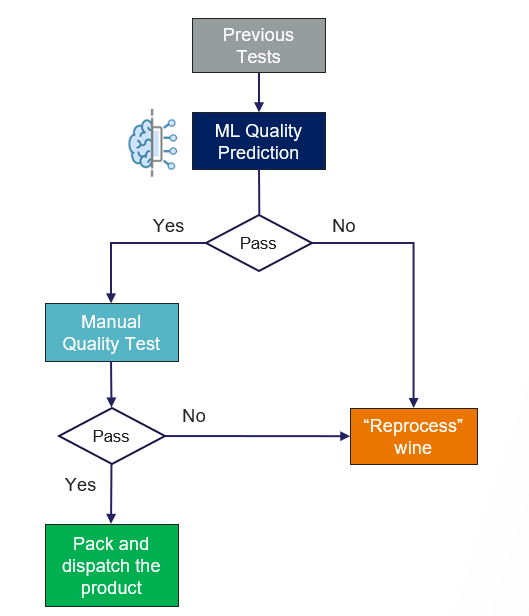
New workflow using the Profiling capability.
Optimizing the production line to fail early
The order of the tests in a production line is normally defined by different business characteristics, such as stage of the product, time reduction, equipment optimization, the frequency of failing a specific test, physical limitations, and others. On top of the traditional variables, SampleManager software uses machine learning to anticipate the future outcomes of tests that will help the production engineers to optimize even further the production line.
In a wine production facility, the result of the “Quality Test” is of utmost importance. The laboratory has great flexibility and control over the testing process, so they could use the Profiling capability to redefine the order of the standard tests conducted to a wine sample.
First, they could calculate the model performance for each of the individual tests they perform.
![]() Evaluating the predictive power of the pH test. |
![]() Evaluating the predictive power of the alcohol test. |
After trying all the tests individually, the laboratory would see that some tests (such as pH) have an extremely low predictive power of the final “Quality Test,” but there are other tests (like “alcohol”) give more information. They would notice that using just one test as a predictor for the “Quality Test” was not enough, as the metrics (sensitivity and specificity) of the model are not sufficient for their requirements.
Secondly, the laboratory could experiment with a set of tests to evaluate their predictive power. In this example, the lab concluded that grouping various tests in three stages could provide valuable information to make the prediction. With such insight, the laboratory modified its standard testing in a three staged workflow:
- Stage 1: conduct alcohol, density, and chlorides. Predict the quality of the wine; if it passes, continue to Stage 2 otherwise fail early.
- Stage 2: Sum volatile acidity, residual sugar, sulphates, total sulfur dioxide and citric acid to the tests performed in Stage 1. Predict the quality of the wine; if it passes, continue to Stage 3 otherwise fail early.
- Stage 3: Finalize the rest of the tests and predict the quality of the wine. If it passes, continue to test the “Quality test” otherwise fail early.
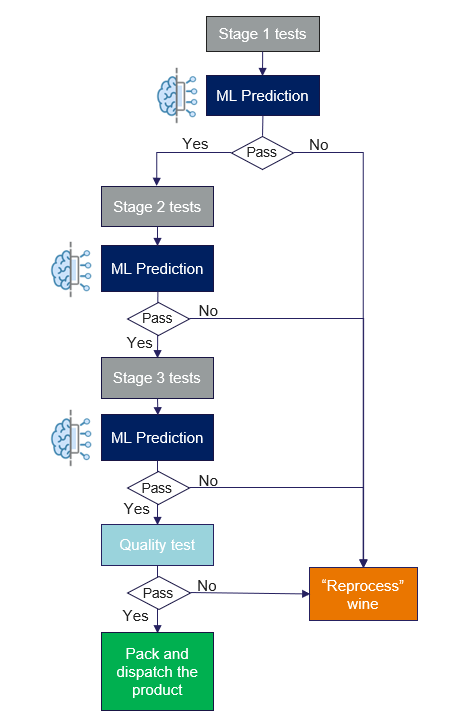
Processes optimized to fail early using the Profiling capability.
Profiling improves production efficiency and eliminates unnecessary testing
The Profiling capability of the Data Analytics Solutions has many potential applications. In wine quality testing, predicting the result of the “Quality test” at various stages of the production line using the Profiling capability could help a laboratory optimize its testing process by reducing the need of unnecessary testing and its associated resources.
To learn more about the Profiling capabilities available in SampleManager LIMS software, visit our Data Analytics Solutions. website.
Leave a Reply